The Digitalization of QA in Manufacturing
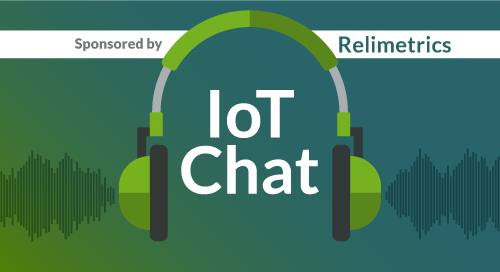
A majority of manufacturers still rely on manual inspections for sorting, tracking and inspecting items. But these efforts are prone to error, resulting in high rework, downtime and unsatisfied customers.
In addition, recent disruptions in the supply chain such as COVID-19 has companies looking for ways to automate QA. In this podcast featuring Kemal Levi, Founder and CEO of Relimetrics, we discuss the changing demand for QA automation and the use of AI to keep up with those demands. Relimetrics helps companies cut costs and improve productivity by automating inspections and using digitized quality data.
Just us as we discuss:
- The challenges facing QA manufacturing teams today
- How AI is being implemented to address the changing landscape
- How the data capture from QA automation can be used to improve efficiency
- What the future of QA automation looks like and how to prepare
Transcript
Kemal Levi: The overall goal in QA automation is the full digitalization of QA. The issue at the moment is that existing quality automation systems are insufficient.
Kenton Williston: That was Kemal Levi, the founder and CEO of Relimetrics. And I’m Kenton Williston, the editor-in-chief of insight dot tech. Every episode on the IoT Chat, I talk to industry experts about the issues that matter most to consultants, systems integrators, and end users. Today I’m talking to Kemal about ways AI is transforming quality control, and how companies can move to fully-automated quality assurance. So, Kemal, I’d like to welcome you to the show.
Kemal Levi: Thank you.
Kenton Williston: Can you tell me about Relimetrics and your role there?
Kemal Levi: Absolutely. My name is Kemal Levi. I’m the Founder and CEO for Relimetrics. At Relimetrics we are enabling the fourth industrial revolution by making quality inspections easier, smarter, and insightful. We enable our customers to digitize visual inspections using AI-based mission vision, and they can do this without writing a single line of code, and they can use this data together with a machine and process data to close the loop in their manufacturing and improve their overall process efficiency.
Kenton Williston: Excellent. On that point, what do you see as being the key challenges? And I guess really maybe the hidden question here is not just what do you see as challenges to quality assurance, but what prompted you to start this company, and what are the problems you’re trying to address?
Kemal Levi: Well, we started our journey as a systems integrator, and we actually did a lot of shop floor work with our customers, helping them implement smart camera technology on their shop floors. We had the chance to really see recurring pain points on the shop floor, particularly related to basically camera-detection accuracy. Today the detection accuracy attained with smart cameras is not sufficient for our customers to really be able to fully digitize their lines. They have a lot of concerns in digitizing their quality assurance, so they need to have quality operators in these lines to weed out false detections. Last week was the thing—the automotive OEM, the quality manager there just made a joke saying that, before it used to be just humans, now it’s humans plus smart cameras. So, to such an ecosystem we basically brought Relimetrics technology that really paves the way for a full digitization of quality assurance in a production floor.
Kenton Williston: So that’s really interesting. And I’m wondering, based on what I’m hearing, it sounds like there’s some long-standing challenges that you’re looking to address. And I’m wondering if there’s anything new in this arena, and ways that today’s challenges differ from the past?
Kemal Levi: With increased customization experienced, what we see is that neither existing QA staff, nor QA solutions are able to keep up with high production variability on the shop floor. So, as I just mentioned, the detection of defects is not sufficiently accurate, and the process control is not optimized. So this is leading to high rework and scrap costs, downtime, and also customer complaints. And, furthermore, manual crane intervention is required to weed out false detections. As a result, like today, manufacturers are actually still relying on human inspections. This is mainly due to actually the poor performance of existing quality automation systems.
So, with basically quality inspections becoming increasingly more complex, what we see is there’s really a push among manufacturers to think about solutions, to help them to digitize their quality assurance processes. What we see is that, today, a large percentage of manufacturers in the USA are still relying on manual inspections. We carried out a study with ABI Research back in November, and what we have basically found out is that only 7% of manufacturers have a fully automated quality assurance process.
Kenton Williston: Got it. So it sounds like there’s a combination of factors here. So, one is the overall effectiveness and accuracy of QA systems—both from the perspective of the existing digital system not performing up to requirements that the manufacturers have, and then of course human beings are obviously fallible; it’s easy for us to miss things. I would say it sounds like there is a cost element, because of course all this labor is pretty costly, and could be better deployed doing things that humans are really good at. And then the third is the flexibility with the custom manufacturing. And presumably the continuing push towards evermore efficient, just-in-time models is also creating a real bottleneck there in the QA process.
Kemal Levi: Exactly. Completely agree with you. This is well stated, well summarized.
Kenton Williston: Excellent. And one thing I’d love to talk about is, of course, the last year has really changed everything about manufacturing. For example, there have just been tremendous disruptions to supply chains, and a great deal of difficulty from the manufacturer perspective of predicting what markets we’re going to need. Like, I just recently bought a house, so I’ve been very keenly interested in the stories about the prices of lumber. And it’s just gone crazy, right? Everybody hoarded it for a while, and the price has shot up; and now it’s shooting down and people are losing tons of money. So it’s become very difficult in this strange year we’ve gone through for manufacturers to know what to do. And so how have you seen this change the demand for QA automation?
Kemal Levi: Over the past few years the demand for software, in general, to automate industrial processes and make them smarter has been in high demand. Today, with COVID challenges, this demand has surged, as companies are looking for ways to protect their employees, and bring as much automation and efficiency to their manufacturing processes to avoid disruptions. So in today’s climate things are improving, but still manufacturing is hit quite a bit—supply chains are disrupted. There’s definitely recovery, but I think we can confidently say that supply chains are still disrupted due to the viral pandemic. So companies are looking for automation solutions that are deployable quickly.
Now inspections by human operators are still a standard in most manufacturing environments. So, as a result, companies are looking at ways to automate those, or have human inspectors operate remotely. So, overall looking at basically the past year, there has really been a surge in demand for quality automation solutions. So we have seen a spike in our sales, and overall we have also seen an awareness on our manufacturers to actually automate their quality assurance processes. So today when we go to a manufacturer and explain to them why they should buy the Relimetrics solution, they are keen to make basically a purchase—whether it’s Relimetrics or some other quality automation provider—they are keen on making the move, because they are certain that this is actually necessary for them to be able to stay competitive in this post-pandemic era.
Kenton Williston: That all makes sense. And one thing I hadn’t thought about before that you mentioned which is really interesting is the idea of doing some of this work with human assistance, but remote human assistance—and then of course things that are fully automated. I would think, in both of these cases, AI would be pretty important—either to do the job just by itself, or to be able to provide remote operators with a more streamlined, summarized view of what’s happening, rather than having to replicate what they would be doing in person. So, how do you see AI factoring into all of these changes?
Kemal Levi: So, there has been traditional computer vision being implemented on shop floors. Now traditional computer vision has been failing in adapting to high production variability use cases. As I stated earlier, this is presenting a big challenge, with increasing quest for customization in manufacturing. Now, the advantage of using AI in addition to a traditional computer vision—like boosting basically computer vision with AI—allows for algorithms to quickly adapt learning to new parts and configurations, and overall any reconfiguring is done much faster. So this is really presenting basically opportunities to reduce downtime associated with the reconfiguring of a quality automation solution. So the adaptability of an AI-based solution is way more adaptable than a traditional computer vision–based solution.
Kenton Williston: Right. And that’s presumably because the traditional solutions would be based around complex algorithms that would be custom tailored to whatever it was you were trying to inspect. So you would have to do very specific thinking about, say, for example, if you were looking for pits, or scars, or whatever in the product—if you built a system to do that, it would only be really good at doing that one task. And if you even changed, say, the size and shape of the objects you were looking at, that algorithm might break. Whereas AI, you can more rapidly update. Is that a good summary?
Kemal Levi: Plus, I think an important thing to note is that with basically rule-based algorithms, you have to show the system every possible configuration. And that is impossible with the sheer amount of customization experience today in manufacturing. AI has an ability to adapt faster to different configurations, different products, because you don’t need to have the system learn every configuration. So, for example, let’s take the work that we are doing at HP today, where we inspect different HP server families. So every product that we inspect is different. This just by itself is actually presenting a big challenge. But there’s even a bigger challenge, because there are also variations in components that are going into each server. There are vendor-to-vendor variations. So with the AI, you have basically an algorithm that is able to better adapt to these differences in configurations. Whereas in the case of traditional computer vision you need to teach the system every possible configuration.
Kenton Williston: Got it. That makes sense. I am curious, though, of course—everyone’s talking about AI these days. You can hardly go anywhere in the tech sector without hearing about it. So, what is Relimetrics doing that is different when it comes to quality assurance, and how does your approach to AI differ from whatever else is out there?
Kemal Levi: In summary, we are changing the paradigm of inflexible part use and reconfigure quality automation systems with smart, connected, and autonomous QA. So we are truly enabling a zero-defect manufacturing. So our product is an industrial-grade framework for anyone without any coding or deep-learning expertise to perform AI-based mission vision and quickly deploy train models inline for real-time inspection on the shop floor. And they can also scale this across different inspection sites. So we enable manufacturers to perform AI-based machine vision without constantly worrying about retraining their models offline each time they have new scenarios or configurations in their production. We also differ from our competitors with guaranteed detection accuracy and overall faster time-to-value on the shelf floor.
Kenton Williston: Interesting. It sounds like one of the big challenges that companies are facing in deploying advanced AI-based QA automation is just the difficulty of deploying it. So can you tell me a little bit more about what the challenges are there, and how are you overcoming those challenges?
Kemal Levi: Together with ABI Research, we developed a maturity model that articulates different stages of quality automation maturity as a company moves from manual to fully automated quality assurance inspections. Now this maturity model helps companies assess where they are today, and what they need to do to move up the automation-maturity curve. Now, based on this maturity model and market assessment, there is still a very large proportion of manufacturers at the very beginning stage of automation. Around one-sixth basically still have quality inspection processes mostly being conducted in a manual process. Now, that said, adoption of QA automation is expected to accelerate rapidly. Most of our customers are highlighting QA automation and robotics integrated, also quality automation as investment priorities over the next two years. Now, from my perspective, ease of use and integration are critical for QA-automation adoption. And this is a recurring issue with quality automation systems. They have required complex integration services over the past decade. I mean it’s—as a result for adoption to actually be accelerated, there’s need for systems that are easy to use and also easy to integrate.
Kenton Williston: Got it. I want to come back to those points about the ease of use, the ease of integration. But before I do that, I do want to come back to another point you made, which is about the level of performance you’re offering with a guaranteed rate of accuracy. How are you able to offer that when other folks are not?
Kemal Levi: There are just a number of things that we do differently with respect to competitors. So, first of all, one key advantage of Relimetrics’ solution is its ability to provide the customers with inline retraining. So, using Relimetrics’ technology, customers can easily monitor what is happening in their production line. And if there are any disputes by the quality operator, or if there are any false detections, they have basically an easy-to-use interface managed centrally by admins who basically have the authority to check whether something is being correctly disputed or not—to basically verify if the disputes are correct or not. And then, if there’s a correct dispute, they can actually revise the training algorithm. So they can retrain algorithms, and using this inline retraining technology, we are able to assure our customers with over a 99.9% detection accuracy across all the components we inspect.
Kenton Williston: Wow, that’s pretty cool. And it sounds like a key part of how you’re able to do that is actually related to the ease of deployment and ease of use of your tool. That’s actually, it turns out, critical to the accuracy as well. So I want to talk a little bit more about that. I would like to hear more about what it is you’re doing exactly that makes your tool easier to use. And, also, the point you mentioned about integration—can you tell me a little bit also about how you integrate with other third-party systems, and why this is important?
Kemal Levi: Well, I mean, I think one advantage of working with Relimetrics, from a manufacturer’s point of view, is that we really understand their pain points, because we started our journey as a systems integrator. We are not the typical software provider who doesn’t really understand what really happens basically on the shop floor. So we are not just looking at things from a software perspective. We also understand the hardware requirements on the shop floor as well.
Now, technology-wise, Relimetrics is use case agnostic—our technology is not limited to manufacturing, let’s say, how many use cases. But when it comes to manufacturing and assembly, we really truly understand the pain points of our customers. So we do know that what really matters to our customers is whether they are going to be able to integrate our solution with different hardware, and also with their shuffler. So this is an inline solution. It is not a standalone solution. It is not an R&D solution. So, at the end of the day, it has to be fully integrated.
We do provide the customer with a full, end-to-end solution that integrates with their lines. It is a fact, that for maximum savings to be provided to a customer, quality automation solutions have to be integrated with other software applications used by the manufacturer—such as manufacturing execution systems and enterprise resource planning systems. This is the only way to really be able to provide the staff with an understanding of their production lines in real time.
How do we integrate with these third-party systems? We do this with fully standardized interfaces. We utilize our own software product, called Node Editor, which eases the pain of systems integration on the shuffler, and enables anyone to be able to quickly integrate our solution with other shuffler software, without writing a single line of code. So this really eases the pain of integration for our customers.
And we also provide the customer with different hardware integration options as well. So this ranges all the way from an industrial-grade camera, to a gantry robot, or a robotic-integrated solution. So we have all the required interfaces, for example, for robotic communication as well. So one software can be used across different use cases for both training and also quality monitoring purposes; it can also do data analytics. It also gives the customer the ability to control different hardware, to manipulate an acquisition, a data-acquisition system.
Kenton Williston: So one thing that stands out to me there in all of what you just said is that there’s going to be a lot of necessity for real-time data. So, obviously, if you’re doing something like controlling a robot, for example, that’s working on an assembly line, or something like that, you need to have any of the control loop happening in real time. Presumably there are also other areas where it’s important to do all those communications in real time. So I’d love to hear more about what those use cases are, and really, even more broadly beyond that, what you see as the best ways to use data generally that’s coming out of these QA automation systems to improve the overall performance and efficiency of a manufacturing line.
Kemal Levi: Well, one: using machine learning for managing a production process like quality assurance—a key challenge to be sold is the amount of data produced by the cameras for inspection. So, for example, let’s take our implementation with HPE and Foxconn. We have a total of seven industrial-grade cameras to capture extremely detailed images of every server. So these cameras generate quite a large load of bytes, of image data per second. It would be impractical to transfer that data via internal or external networks to be processed on remote service, because the latency would be too high and the networks will be overloaded with these data volumes. So, therefore, I mean, we deploy our software on Edge systems, such as HPE Converged Edge Systems, for example. So these are robot compact systems delivering enterprise, great IT capabilities at the Edge, in close proximity to the data source.
Kenton Williston: So I think what I’m hearing is that there’s a couple different elements of data utilization. So, one is within your system itself—being able to process the video feeds at the Edge, make decisions, and then provide information about what that QA system is observing in real time. Then the other piece of that is being able to take the output from your QA system and use that to improve the overall efficiency and performance of what’s happening on the shop floor. So I’d like to hear a little bit more about that second part—of how that data can be used to inform the bigger picture of operations.
Kemal Levi: Being able to monitor the data is really critical for the success of our customers. Now, overall, what we offer the customers is a holistic approach to data—where machine and process data are collected and correlated against digitized quality data. This approach Relimetrics is offering combines automated defect and geometry analysis with data-correlation approaches to prevent quality drifts that happen during production. And, by automating inspections and using the digitized quality data, we help companies cut costs and improve productivity. So far, with our existing customers, we have showcased that overall productivity improvements range between 50% to 80%.
Kenton Williston: Well, that’s a pretty impressive jump. What I’d love to hear, if you go a little further there, is if you can talk to some specific use cases that you’ve been involved in in different industries, and what exactly you were able to do to help your customers succeed.
Kemal Levi: Well, we have many use cases we address with the Relimetrics software—the same software product. So what really excites me is the applicability of the same software package across different use cases. So, in a way, I mean, Relimetrics is an industrial-grade Adobe Photoshop, which can be used by anyone to train AI-based machine vision algorithms without writing a single line of code. So there’s quite a bit of a mashup of the work we are doing with HPE in the media. We are the global video-analytics vendor of HPE, helping them with digitizing QA of their servers across their contract-manufacturer ecosystem. So we have been working with HPE for quite some time now.
So the very first implementation that we took was at Foxconn factory in Europe, where we helped Foxconn with full digitalization of QA across different HPE server families. So this is very exciting work, which is scaling at the moment globally to other contract manufacturers of HPE. We are very active in the electronics assembly at the moment. In addition to the work that we do in electronics assembly, we are also working with Lockheed and the aerospace industry—helping them with very exciting advanced manufacturing use cases, where we are actually enabling AI-driven manufacturing. Which is a very exciting use case, because there we are really going beyond quality automation—we are actually using the digital twin, which is utilized to guide autonomous manufacturing activities—whether it’s, let’s say, drilling or riveting. And we are quite active in the energy industry as well. Today we are working with Siemens Gamesa helping them with digitization of phased-array ultrasound data using the real metric software. So this is an exciting use case for us as well, where we showcase the applicability of Relimetrics software to a different image modality.
We are also working with customers where Relimetrics software is being adapted to detecting anomalies in X-ray images. This is really exciting because many of these customers already have existing X-ray systems, and they either have quality operators reading anomalies in the images, or an existing, traditional computer vision–based automated optical inspection system assessing the quality of the images. But these are providing the customers with a poor detection accuracy, so they are bringing in Relimetrics technology to boost the detection accuracy, and also enable them to really think about full digitalization of QA.
Kenton Williston: Well, that’s really cool. And, I have to say, this is very interesting to get this picture into everything that’s happening, and how different what you’re doing is from what people have been doing in the past—even with everything that traditional computer vision systems can do, it just seems like a huge step change here, and that makes me think about what might be coming in the future. So what do you see coming, in, let’s say, the next 10 years in QA automation, and how are you preparing for that?
Kemal Levi: The overall goal in QA automation is the full digitalization of QA. The issue at the moment is that existing quality automation systems are insufficient to think about full digitalization of QA. So I think in 10 years it’s going to be: QA automation will be fully digitized, thanks to AI. There’s also another angle to the story is that is, for example, in the case of assembly—it is the automation of the assembly using robots that basically also incorporate AI in a very intelligent way. They are able to actually perform the assembly, and also run the inspection on the parts that basically they assembled. So I think the next 10 years in QA automation are actually going to be drastically different than where we are today at the moment. Definitely, the push is for AI-driven manufacturing at the moment, and Relimetrics-like technologies will be enablers for this type of robotic system to succeed.
Kenton Williston: Got it. One key thing that we really haven’t touched on that I think is important to surface here. You talked a little bit about how your systems run at the Edge, often on HP servers. Presumably, underneath the hood there you’ve got some Intel technology, and I’m curious how your partnership with Intel has added value to your system. And, also, as you’re talking about these things, looking forward, how you see that relationship with Intel evolving and helping you go forward to the future you described.
Kemal Levi: Well, I think overall our success is made possible by using advanced Intel Edge computing technologies. So, specifically, we today use servers based on powerful Intel Xeon processors, and Intel provides the software and architecture that enables Relimetrics to make the best use of basically CPUs. So this technology is critical to our quality assurance solution, because it enables us to run computationally intensive calculations at high speed.
Kenton Williston: And, presumably, in terms of looking forward, you’ve got a lot staked on Intel’s roadmap. And they just released this year, for example, the Ice Lake platform, which has a lot of AI accelerators. And I would imagine you’re excited about using those.
Kemal Levi: I am very excited about, actually, what Intel is currently working on. So another thing of being an Intel-certified solution is the potential business opportunities that Intel also brings to us. So, on the marketing side, this is definitely just a very powerful way to showcase that Relimetrics solution is a certified solution. So that’s one advantage. But another advantage is Intel teams have been very open in helping us out and bringing us in front of their customers—big accounts of Intel. So I’m really excited about actually the work they are currently doing together with us. So I think there are really great opportunities for us to collaborate on.
Kenton Williston: Great. Well, I can’t wait to see where things go next with that. As we’re getting close to the end of our time together, are there any key takeaways you’d like to leave with our audience?
Kemal Levi: It’s really important for our audience to really be aware that AI is coming very strong. I think the pace of change in the world is at a much more accelerated pace than it used to be before, and I think there are those who still think that, for many use cases, AI-based mission vision is really not going to be successful. And we experienced this—many times there are basically questions posed by academics, there are many adopts. But we are all proving them wrong. AI-based mission vision really operates at a stellar performance—way better than traditional computer vision today. I mean, this is—I mean, these are the facts on the ground, and I think overall where we are heading is going to be the full digitalization of QA. So companies should really act fast in making sure that they really operate in an efficient way, and think about going basically to an advanced level in their quality automation journey.
Kenton Williston: Excellent. Well, I can’t wait to see all of this transpire, and, until then, I just want to say, thanks again, Kemal, for joining us.
Kemal Levi: Thank you very much for your time.
Kenton Williston: And thanks to our listeners for joining us. To keep up with the latest from Relimetrics, follow them on Twitter @relimetrics and on LinkedIn at linkedin.com/company/relimetrics
If you enjoyed listening, please support us by subscribing and rating us on your favorite podcast app.
This has been the IoT Chat. We’ll be back next time with more ideas from industry leaders at the forefront of IoT design.
The preceding transcript is provided to ensure accessibility and is intended to accurately capture an informal conversation. The transcript may contain improper uses of trademarked terms and as such should not be used for any other purposes. For more information, please see the Intel® trademark information.