Is Time-Series AI the Next Megatrend for Industry 4.0?
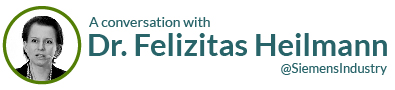
Think machine vision is powerful? Wait until you see what time-series AI can do! Ideal for analyzing non-optical data such as voltage and temperature signals, time-series AI is poised to play an outsize role in the factory of the future.
In this podcast, we explore the possibilities with Dr. Felizitas Heilmann, Head of Product Line for Embedded Industrial Computing at Siemens. As one of the biggest players in industrial automation, Siemens has already built considerable expertise in this burgeoning technology. Join us as we discuss:
- The most promising applications for time-series AI
- How to deploy this new technology into legacy infrastructure
- How systems integrators and machine builders can successfully deploy time-series AI
Related Content
To learn more about machine vision, read Q&A: Is Time-Series AI the Next Megatrend for Industry 4.0?. For the latest innovations from Siemens follow them on Twitter at @SiemensIndustry.
Transcript
Kenton Williston: Welcome to the IoT Chat, where industry experts examine the technology and business trends that matter for IoT developers, systems integrators, and end users. I’m Kenton Williston, the Editor-in-Chief of insight.tech. Today I’m talking trends in industrial AI for 2021 and beyond with Dr. Felizitas Heimann, Head of Product Line for Embedded Industrial Computing at Siemens. Among other things, we’ll explain why time series AI will play an outsized role in the factory of the future. And we’ll look at the ways systems integrators and machine builders can set themselves up for success in the increasingly complex world of AI, deep learning, and machine learning. There is so much to discuss, so let’s get to it. So, Felizitas, welcome to the show.
Dr. Felizitas Heimann: Hello, Kenton. It’s a pleasure.
Kenton Williston: So, tell me a little bit about your role at Siemens.
Dr. Felizitas Heimann: Well, I am responsible for our product line of embedded industrial computing, which means basically our box-and-panel portfolio. That means leading the product management, and also leading the technical project management, which is a very nice combination of responsibilities because there’s so much you can do and decide on and execute. So it’s really fun, and it’s currently an amazing situation to be in, in factory automation, because there’s so much going on.
Kenton Williston: That’s great. And with that, I’d love to just jump right into the topic of today’s conversation—because there is so much to talk about—and talk about what’s happening in AI. So, what do you see as the biggest trends in shop floor AI for 2021?
Dr. Felizitas Heimann: So, when thinking about the current state of industrial AI, I’m thinking of a hype cycle. I think about it in a positive way, because the best part of a hype cycle is always the valley of disillusionment, where, constantly, real applications are starting to pop up and be spread and multiplied—that’s definitely the sweet spot of any innovation coming through. But you were asking about the nearby trends, and I see three of them in the near future. First, concerning applications and use cases. I mean, what is closest to being applied on a big scale is probably AI-assisted machine vision—for example, for optical quality inspection—and that’s for several reasons. AI-based image analysis is already quite well understood, it’s comparatively easy to apply, and the quality of results is easier to control than for other AI applications. As you’re dealing with one kind of data source only, especially in industrial environments, you cannot allow for any unnoticed errors.
So being able to control the quality of your tool is a crucial feature. And that’s why I assume here we’re going to see the fastest progress in terms of spreading applications on a large scale. Second, progress in hardware. We’ll see more and more dedicated or adapted shop floor AI hardware appearing—mainly PC-based, as almost all industrial microprocessor companies have started to offer AI accelerators that are now getting applied in devices like industrial PCs. And that’s one important step to enable the deployment and the application of AI models on the shop floor—also for brownfield applications.
And third—and this is a very important one—shop floor computing architecture. We’re seeing IT/OT architectures at scale recently, where the most prominent example may be industrial Edge computing. That means connecting the shop through assets to central management; providing additional computing power resources; but, compared to cloud computing, keeping as much performance as necessary on the Edge—so, meaning close to the machine or the line. And due to the nature of how AI works, this is a mandatory prerequisite. So these would be the biggest trends that I would see in the very near future, where AI will be supported and will start to make progress.
Kenton Williston: So, I want to come back to one of the first things you said, which was about the types of AI that are being deployed right now. So, like you said, it’s really the visual AI that has taken off. And I think there’s something of an irony there in that, from an office perspective, you might think that vision systems would be some of the most complicated and difficult to deploy, because vision is such a complicated process from a human being’s perspective. But, from a mathematical perspective, in some ways it’s one of the easier sorts of AI, because it’s relatively straightforward, like you said—it’s a uniform kind of data that you’re dealing with.
And, I think, importantly, it’s something that, although it’s a complex process, humans intuitively understand vision. And there’s many other kinds of AI, and particularly time series AI, that I think are maybe not as intuitive to judge the results, but I think are very important. And I want to talk a little bit about that today. So can you tell me a little bit about what time series AI is? And why you think it’s going to play such a large role in the smart factory.
Dr. Felizitas Heimann: I can, and you put it totally right. Especially in AI, there is a phenomenon: what looks easy is the most complicated thing; what looks complicated in the beginning, like machine vision, is eventually even the easier thing. Why I believe that time series is actually the most rewarding use case of AI in the long term is the ubiquitous availability of the data available, and the massive unused potential to you to gather insights out of it—I mean, non-optical data are everywhere. In a line you have continuous inflow of data from different sources. For example, you can think of electrical data like current voltage spikes, temperature data, mechanical-like vibration, expansion, flow speed, pressure. There’s a massive unused potential in using these data.
Kenton Williston: Yeah, absolutely. And something that I actually have some experience with myself, and at much earlier, earlier stages of my career (I hate to think about its numbering in decades now, how long ago this was). But there’s so many different kinds of systems—whether the mechanical systems, chemical systems, whatever—where there’s just a lot of action happening that can’t really be evaluated by vision systems. So, reflecting on some of my ancient experience—for example, I used to work at an engineering firm that designed petrochemical plants, and so there’d be all sorts of chemistry happening and different flows happening, different pipes that needed to be measured, what kind of temperature and velocity, and is there cavitation happening—or any of these sort of considerations.
And, at the time—and this is, again, getting to be decades ago—everything was done in a very simplistic fashion. It was obvious to me then, and even more obvious to me now, that AI is a little bit more of a viable concept that, with some more advanced algorithms, the efficiency of the plant could be improved, the likelihoods of failures could be reduced, and so forth. So, could you highlight for me some of the examples that you have in mind when you think of where time series AI could be applied?
Dr. Felizitas Heimann: Yeah. We have a great example in one of our own plants, and that makes it of course easier to talk about it as well. One example of one of our own manufacturing sites: out of a data analysis of a whole PCB assembly line, the algorithm that had been developed has been able to judge, with which confidence an extra X-ray analysis of a PCB board is necessary, or not, in terms of quality control. With this AI solution, the purchasing of an additional X-ray could be avoided, so saving several hundred kilo euros while maintaining the same level of quality. There are many examples that are going in this direction. The main challenge for the time series application is: the more complex the input gets, the less easy it becomes to understand the model and the dependencies. So, like you were saying with your petrochemical facility, you can have a vast amount of sensors, so it becomes more important to test the model, and also to permanently monitor its KPIs if the model is still acting as it is supposed to be.
Kenton Williston: Yeah, for sure. And I think one of the big challenges there, is data that’s maybe not so intuitively understandable to a human being, unlike an image or a video. But it’s also many different sources of data, that you might want to combine to make some assessment—like vibration in the machinery or the electrical load, or all kinds of different things you might want to monitor simultaneously. And reaching an intuitive understanding of: what do all these things combined mean? is quite difficult. So it’s a perfect opportunity to ask a machine to do the job. But it also means that it’s something that’s not immediately obvious how you would do that.
Dr. Felizitas Heimann: This will require a long way to go. Honestly, there is a rough road ahead of anyone who intends to apply that.
Kenton Williston: And I think also it brings to mind, to me, something we’ve talked a lot about on the insight.tech program, which is just the generational shifts. So, I know here in the US, and there in Germany as well, there’s a large demographic bubble happening, where this baby boomer–sort of generation is very quickly retiring. So you have a lot of human expertise that’s very quickly leaving all sorts of industries, and a lot of accumulated wisdom as to how the different equipment in the plant operates and how to understand when there are problems, just by years and years and years of experience. So I think it’s pretty essential to start making some AI models that can capture some of this expertise in a way that will allow the next generation of the workforce to be successful.
Dr. Felizitas Heimann: That’s, of course, true on the one side. On the other hand, I strongly believe that humans will be required in the future as well, and experienced humans who know what the models are doing, and are not just handling black boxes but have a deep understanding of what’s actually going on in their line to be able to judge.
Kenton Williston: For sure. And that leads me to another topic I wanted to discuss with you, which is on this subject of having human beings who understand what’s happening. I think one of the other big challenges that I see—it’s both a challenge and, I suppose, a benefit of the way things are evolving—is there’s this huge trend towards IT/OT convergence, and I think that the benefits of this are pretty powerful from the enterprise side. For example, you can gain so much better visibility and understanding of what’s happening in your facilities. And from the OT side you can get access to just these amazing resources—the data centers and clouds and things like that. But it’s challenging, I think, for this convergence to happen, because you do have two worlds coming together that don’t necessarily understand each other very well, and then you add AI into the mix and it gets really complicated. So how do you see this IT/OT convergence factoring into the evolving role of AI?
Dr. Felizitas Heimann: So, I would like to narrow down the benefit of IT technologies in the OT world to just a simple statement, and that’s: increase options while improving resource efficiency. And, as you have just mentioned, both sides have a tremendous potential in the combination of both. And if we’re bringing that back to our AI topic, especially for a neural network AI application, you have to differentiate between two kinds of activities. Namely, training a model with historical data, where the result is already known; and , which means using the model to judge new data sets. And the training accounts for a small percentage of the overall usage time of the model only, but during that limited time it requires orders of magnitude higher computing resources. The inference, on the other hand, is running permanently with a much less, but continuous, performance requirement. So you need to calculate this continuous computing workload for inference, and you run it on a computing process close to where the data are generated.
And, additionally, you plan model training and retraining effort. And you want to assign this either to an on-premise server or somewhere in the IT department, or completely off-site on a cloud server, for example. So one of the core elements of IT/OT convergence from that standpoint is the industrial Edge management—as its core domain is to connect these two layers of computing availability, and making them convenient to use for an industrial automation engineer in a way that he or she does not have to be an IT expert for it. So, otherwise cost-efficient deployment of AI would not be reasonably possible. But that’s only one part of the story.
The IT/OT integration, and especially the industrial Edge, additionally also provides the option of new business models. For example, as a machine builder, as a systems integrator, you might want to offer predictive maintenance of the machines or lines you have sold anywhere in the world, and you might want to use AI on that device to support the customer with information. The industrial Edge device then supports you or your customer to collect and pre-process the necessary data for that, close to the asset that you want to monitor, and helps to transmit it to your central system. So all use cases like this are based on the industrialized availability of IT technologies, and especially convenient and secure availability.
Kenton Williston: Yeah, absolutely. And, of course, one of the things that I think is really important here is what you mentioned earlier in your introduction—how much the computing hardware has changed. And part of what’s made all of this possible is the fact that IT-style compute hardware has become so much more powerful—is having so much more built-in AI capability. So I’d love to hear a little bit more about how you think these sorts of advances in industrial computing hardware have changed the AI landscape.
Dr. Felizitas Heimann: To answer that, maybe we have to jump in a little bit into what AI is about in terms of computing. And due to the nature of the mathematical model of the neural network computing behind it, it’s all about parallelization, computing speed, and accuracy. And for the parallelization, you’re talking about the numbers of computing cores. And for the computing accuracy, you need to consider which kinds of data you need to handle. , because vision usually works with integer values, because the pixel input from the image is usually normalized in the same manner by the RGB value spectrum.
So all calculations can happen in the integer range and can be ideally processed. We have the high number of compute cores of a GPU. Time series, instead, can come from different data, from different sensor input, that at one point in time can have a dynamic range that differs from the range in the next period of time. Also, due to these different ranges of accuracy that the individual sensors provide, your pre-processing and your normalizing efforts get much more. So with GPU computing technology, you will probably not be able to handle that at all times. For multisensory time series applications, on the other hand, data input means handling dynamic and different data ranges.
So all input data need to be permanently normalized as a pre-processing step, making the inference calculation much more complex—to handle the accuracy integer values might not be sufficient. So CPU-based, multi-core computing resources are what you will go for for these kinds of use cases—you will find them in industrial computers. To scale efficiently, you need to choose your hardware based on two factors: the performance required per single inference, and the necessary number of inferences in a given time. So, coming back to your initial question of what has changed in industrial computing hardware, to choose the right computing hardware you’d want to look into the number of sensors, data rates, sound rate, the normalization technique, and the algorithm to be used, and with which KPIs you intend to control the quality of the model.
To give you a feeling of today’s industrial PC range—our IPC range is currently from dual core Intel Atom up to dual socket 28 core Xeon CPU processor. And our fanless IPCs can be mostly equipped with a small AI accelerator code. And the largest racks can support up to two NVIDIA, like P5 cells and GPU cards. And we are continuously increasing our portfolio to meet the rising demand of more and more AI-specific hardware.
Kenton Williston: That’s great. And, of course, it’s not just the compute hardware that I think is changing and making new possibilities happen, but there’s all sorts of things happening in the networking space as well. It’s been interesting over the last couple of years to just watch this transformation. Again, I think back to the old days from my own experience, and how really isolated any kind of automation might be. I mean, I started my career back in the PLC days, where programming looked more like circuitry. So it was kind of primitive, but the communication between the systems was even more primitive—if it even existed—and you’d have all these different network standards, and it was very expensive to connect things together. And of course that’s changing a lot now—there is a move towards more standardized kinds of networking with technologies like TSN. And then, of course, there’s a lot of excitement, and I think it’s merited, around 5G. So I’m curious what you see as the potential for these new network technologies as we move forward.
Dr. Felizitas Heimann: What we’d expect from 5G on the shop floor in the next period of time? High bandwidth, wireless communication, high local availability, high flexibility. And if I project to what to use that for in the beginning—especially as there are still a lot of brownfield applications—if you think about application of AI, the local 5G will be a quite nice way to equip brownfield—so to connect assets and sensors to an industrial IoT environment.
Kenton Williston: Yeah, absolutely. And I think one of the things that makes that really great is when we think about AI, and you mentioned earlier the importance of having really good compute capabilities at the Edge, because you need to get close to these data sources. One of the reasons for that is because there can be an incredible amount of data that you want to analyze, and that’s particularly true in systems that are driven by visual data. But then when we start thinking about time series AI in particular, there’s many, many examples where the bandwidth is quite a bit lower, and it’s like we’ve been discussing—more a matter that you want to collect a lot of data from different sources and/or you might be able to use a single stack of compute hardware to run analytics for many different pieces of equipment on the shop floor. And I think, in this case, the technologies like 5G will be extremely useful for enabling you to do some sort of a centralized influencing. What do you think about that?
Dr. Felizitas Heimann: Exactly. And there also the great benefit of TSN will come into place when, especially, you want to connect time-sensitive data that was in your network here without a big setup effort. And translated to AI, again, this could be the case when the data inflow to your inference model comes from these distributed sources and requires a high position and reliability on the timing. So I believe both technologies will broaden the opportunities a lot. You have to set up a reliable, real-time processability of data for AI applications.
Kenton Williston: I’m glad you mentioned that real-time aspect—something really critical because, of course, it’s not just a matter of making some conclusions about the data that you’re seeing, but also taking some immediate action in many cases—for example shutting down some machinery before damage occurs. So having that real-time responsiveness is quite critical. And you mentioned the different kinds of compute hardware that Siemens offers and, of course, one of the things that’s been pretty cool here recently—the so-called Elkhart Lake product launch. The Atom 6000 series has some of these really important real-time capabilities built right into it, which really helps support some of these use cases.
Dr. Felizitas Heimann: Yeah. Probably it’s quite easy to hear from that presentation that it’s going to be one of my favorite devices in the future. In any case, our upcoming generation of IPCs will feature incredible opportunities also, especially for AI applications—for any other, of course, as well. But as we’re talking about AI today, we’re doing a lot to have systems that are really matching the use case, considering it from a customer perspective. Especially the Elkhart Lake platform will be really cool.
Kenton Williston: So, as cool as that is, all of this great compute hardware doesn’t really mean very much until you can actually program it and make use of it. And I’d love to hear your perspective on the kinds of platforms and tools systems integrators and machine builders need, to be able to successfully create and deploy time series AI.
Dr. Felizitas Heimann: I have a personal opinion about tools, and that comes from experience. For any tool you have garbage in, garbage out, and this is also true for AI models. So the key initial thing to do right or wrong is the data preparation in the beginning before thinking about any tool, and also to be able to think from the perspective of what the training process will do with your data. And there’s a small anecdote of a true example which highlights that quite well. We had a case where the model capability seemed excellent—100% discovered good from bad training pictures. Unfortunately it could not at all reproduce this in real operation. So, what had happened? All the good sample pictures had been taken at the same time, and—especially—at the same light settings; and the bad samples had been taken any time, under different optical conditions. So, in the end, the machine learning model had not trained itself to identify the defects, but to identify if the light settings were the ones that apparently had resulted in good or bad quality.
So, probably everyone who starts working with this matter falls into these traps at first. And it reminds me very much of the early days of numerical simulation—where everyone was quickly able to generate colorful, seemingly reliable, but totally misleading results. So to be successful is to know what you do, and what you can possibly expect. And for that, the most important tool—we talked about it already—are humans. And for a systems integrator, I would definitely recommend to develop industrial application engineers coming from the automation domain who are familiar with OT requirements, and who want to adopt the new technology, and this is no matter of age.
Kenton Williston: Yeah, absolutely. People who really understand, sort of on an intuitive level, how these systems work. It’s really great, because, like we talked about with vision systems, that’s something that, although there are pitfalls—like you mentioned with the lighting, which is a pretty funny example—it’s sort of intuitive to a human being what the machine is seeing, and then you get into these time series things where it’s voltage or temperature or whatever—it’s not so intuitive. So, very important to have someone who already understands what the data means.
Dr. Felizitas Heimann: Exactly. And I believe in the midterm things will get a little bit more easy for the, say, general automation public to handle because what we will see are more ready-to-use applications evolving. For example, here in Germany—so sorry for the German word—our Mittelstand, so, our small and medium enterprises, who really have a fantastic domain know-how, that are sometimes world leaders in their small domain. I would expect companies like these to deliver really great, highly-specific solutions, and then roll them out on the basis of the big platforms—like for example, our industrial Edge management. And by that, things will get more easy to apply and to handle even if you’re not a machine learning specialist.
Kenton Williston: Yeah, for sure. I think we’ve seen that happen already in the vision space, where now there’s open platforms like Intel OpenVINO toolkit, where you have a lot of existing models that you can use as a starting point, and a lot of community knowledge about how to use those and how to avoid the kind of pitfalls like the lighting pitfall. And I think it’s definitely going to happen that we’ll see the same sort of things happen in other areas in the time series AI as well.
Dr. Felizitas Heimann: Exactly. And both on the hardware and the software level, there’s a great advantage of open architecture. And our industrial computers, for example, they enable the customer to use our devices either with their own software, with third-party software, or to go with our offering—which is continuously growing, as well as part of our industrial Edge ecosystem. And luckily for the AI modeling tools, it’s the same—they’re getting reasonably well exchangeable via standard. So you can sort of let your AI engineers work with their preferred tool set and then port it into your preferred industrial environment, including the operating system.
Kenton Williston: For sure, and that leads me to my next question. You mentioned the Siemens ecosystem, which is very robust, of course—you’re for many decades running one of the world’s leaders in industrial automation. And I think that’s an important thing for machine builders and systems integrators to keep in mind—is what’s already out there, and how they can best leverage this existing infrastructure. So, what do you see as being the critical considerations when it comes to working in these brownfield environments, and leveraging the existing infrastructure when you’re trying to launch some new AI technology?
Dr. Felizitas Heimann: Well, depending on the intended application, of course, you need to start at the assets and field devices level, and especially in brownfield applications—new applications as well—you will have an existing base. And you will have to ask yourself, are you able to collect all the data you need for your use case based on the existing sensors or other information? Or do you have to equip more? And what you will definitely have to invest on also in a brownfield application—there will usually not be the excess headroom in computing capacity to run the inference at the desired speed. So, in most cases, an investment needs to be made to add additional Edge computing performance close to the application, which, luckily, an industrial computer can be easily connected to the system via the standard known connectors or protocols.
Then, especially, our industrial Edge management software enables convenient server connection, remote deployment, and the remote monitoring. And, again, there we take great care to develop it in a way that it blends smoothly into existing environments. And then, of course, what’s planning a new line, the industrial PC resource can of course be counted into the overall performance requirement, and eventually be chosen in a way that the inference can run in parallel with other applications—for example, a local HMI monitoring.
Kenton Williston: That makes sense, and leads me to my final question for you—which is how machine builders and systems integrators can engage with Siemens and your partners, for that matter, to simplify the design process and get these really innovative new time series AI designs out there?
Dr. Felizitas Heimann: Well, there are many ways. For example, we’re building industrial digitalization specialists all over the globe—for AI, for industrial Edge, for new PLM software tools like digital twin, for example. No matter if your current contact to Siemens has supported you on hardware or software topics, , PLM software, or other—he or she can direct you to specialists who can support you either in finding the hardware and software solutions when you just need the suitable components, or even to arrange a consulting service to go on the journey with you together—supporting you with deep industry and domain know-how. And part of the Siemens customer individual offering, for example, can also, for example, be the model monitoring to be aware if parameters start to run away, and also if retraining is needed. And we’re continually enriching our portfolio on the hardware and software side as well. It’s really exciting to see how quickly things are moving in that field currently. And, for a starter, you can check that out at www.siemens.com/pc-based.
Kenton Williston: Well, with that URL, it’s a perfect place for me to end, and give some other places for our listeners to go for additional resources. So, thanks for providing that. And thanks for joining us today, Felizitas. Really appreciate your time.
Dr. Felizitas Heimann: Thanks having me, Kenton
Kenton Williston: And thanks to our listeners for joining us. To keep up with the latest from Siemens, you can follow them on Twitter @siemensindustry, and on LinkedIn at showcase/siemens-industry-us. If you enjoyed listening, please support us by subscribing and rating us on your favorite podcast app. This has been the IoT Chat. We’ll be back next time, with more ideas from industry leaders at the forefront of IoT design.
The preceding transcript is provided to ensure accessibility and is intended to accurately capture an informal conversation. The transcript may contain improper uses of trademarked terms and as such should not be used for any other purposes. For more information, please see the Intel® trademark information.