AI Rides the Rails to Automate Track Inspection
Rail travel is one of the most popular modes of transportation in the world, especially in high-density urban centers where throngs of people pack into trains to commute across town. As you can imagine, sending locomotives and rail cars weighing hundreds or thousands of tons down two steel beams makes ongoing safety inspections the highest priority.
But did you know that most of these inspections, including those of train tracks, are still conducted manually?
That’s right. Most track inspections, even in the U.S., are still performed visually by a human who walks or drives down a track and stops periodically to examine conditions, measure the gauge or distance between rails, and check that track fasteners are properly secured. The time consumed worldwide by this exercise is difficult to comprehend.
With all the recent advances in AI and computer vision technology, automated inspection solutions can replace human inspectors to save time, cut costs, and improve the coverage of visual track inspections. That is, if the AI system is engineered well enough to withstand the rigors of the rails.
AI Inspection Automates Track Safety
Take track fastener inspection, for instance: an AI inspection system comprising an HD camera mounted under the locomotive, AI models that classify the angle of rail fasteners, and an edge computer that can monitor the integrity of every single rail fasteners, then relay abnormal inferences back to an operations center so technicians can be dispatched if necessary (Figure 1).
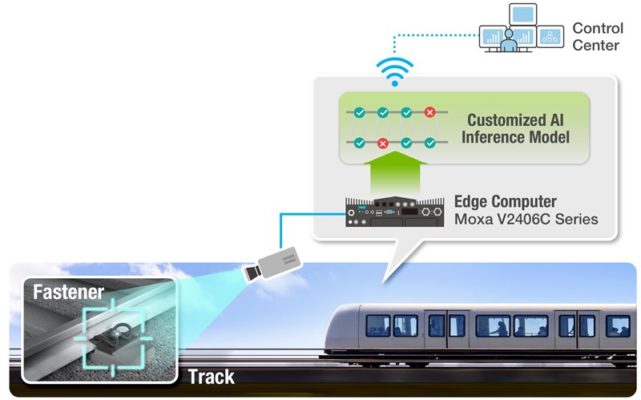
This is far more efficient than sending a human to check intermittent rail fasteners and, best of all, the train carrying the automated inspection system is already headed that way to begin with. And not only does this accurately detect any issues, but it also helps ensure passengers and cargo are traveling on the safest route possible.
Of course, all that sounds much simpler than it really is. A system like this requires a highly accurate, custom AI model; sufficient processing power to run it at the edge; enough efficiency to run on limited resources; and the ability to operate for years in harsh environments in accordance with standards like EN 50155.
Solutions that meet all those requirements don’t just fall off the back of a train.
With all the recent advances in #AI and #ComputerVision #technology, automated inspection solutions can replace human inspectors to save time, cut costs, and improve the coverage of visual track inspections. @MoxaInc via @insightdottech
Eyes on the Rails
Realizing the potential benefits, a train operator in Asia enlisted the help of Moxa Inc., a leading provider in industrial computing and maker of the V2406C Series Multi-WWAN Rail Computer.
The V2406C is an EN 50155:2017- and EN 50121-4-compliant onboard and wayside railway computer designed for heavy-duty data processing tasks that was already deployed in trains as an onboard CCTV and NVR storage and video processing platform. Based on 7th generation Intel® Core™ processor technology, the V2406C has the wide -40ºC to +70ºC temperature support needed to subsist in rolling stock environments for up to 15 years as well as the power efficiency to operate constantly without draining resources.
But to achieve the 90-percent-plus accuracies required by the track fastener use case, the V2406C needed an extra boost. The nudge it needed was delivered via the Intel® Distribution of OpenVINO™ Toolkit and Intel® Movidius™ Vision Processing Unit (VPU) acceleration modules.
The OpenVINO toolkit automatically optimizes AI models developed in frameworks like Caffe, TensorFlow, PyTorch, or MXNet for deployment on a range of Intel silicon, including Movidius VPUs. In the track fastener inspection system, these VPUs were integrated by plugging two acceleration modules featuring them into mPCIe slots on the V2406C Series. The OpenVINO suite was then used to compress a custom track fastener classification algorithm designed by Moxa and a system integrator to fit on the V2406C Series’ 32 GB of onboard DDR4 RAM.
The resulting AI inspection algorithms execute on the Movidius VPUs to identify track fasteners with abnormal angles of greater than 18 degrees in real time, yielding more than 90% classification accuracy.
Back from the Wayside
Today, the train operator logs inferences from the V2406C AI inspection system on two hot-swappable HDD/SSD storage expansion drives, then reviews trip data at an operations center when trains return from their routes. But there are plans to make use of redundant LTE/Wi-Fi connectivity afforded by the V2406C’s two mPCIe wireless expansion slots and four SIM card slots to wirelessly transmit inference outputs and positioning data back to control centers from wayside trains.
By tying this edge intelligence back to a central hub, train operators can convert human inspectors into maintenance technicians who are dispatched only to specific sections of track that require immediate attention. The result will be better track conditions, fewer delays, and lower operational costs, all thanks to automated inspection via the AIoT.
This article was edited by Christina Cardoza, Associate Editorial Director for insight.tech.